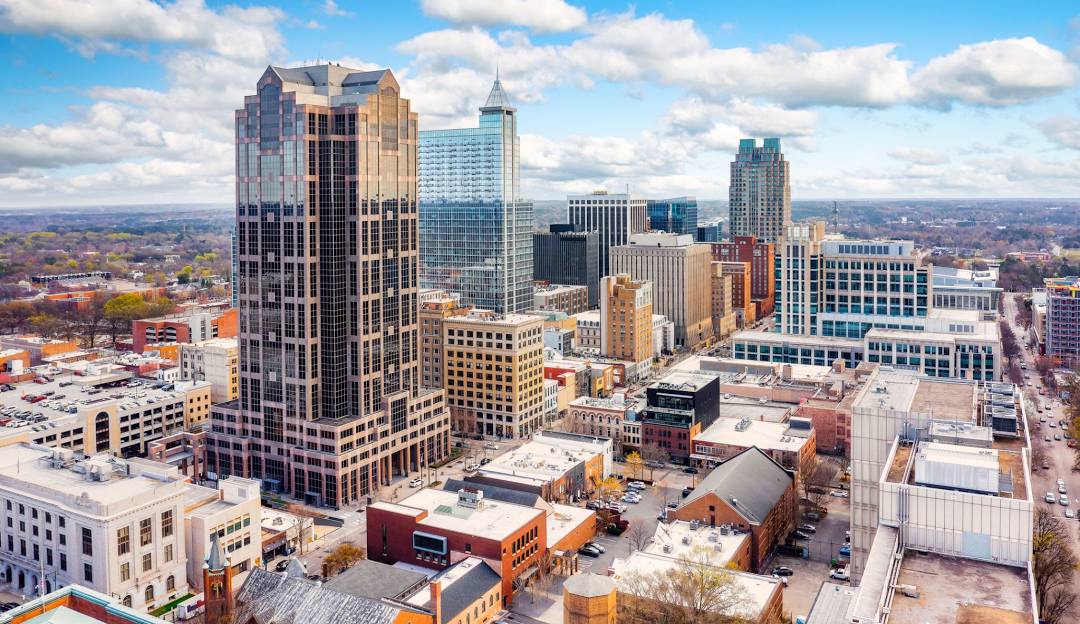
TACPS 2024-OCT In Conjunction with ESWEEK 2024
The 2rd International Workshop on Trustworthy Autonomous Cyber-Physical Systems (TACPS 2024-OCT)
Autonomous cyber-physical Systems are rapidly integrating into our everyday lives. These systems are capable of operating and performing tasks without explicit human intervention to various degrees. Such systems span a diverse array of applications, including, but not limited to, self-driving vehicles, unmanned aerial vehicles, robotic manufacturing systems, autonomous surgical robots, smart home systems, smart farms, autonomous submarines and unmanned space rovers. In a world increasingly reliant on autonomous cyber-physical systems, trustworthiness is not just a topic within academic circles, but also a critical concern in industry. The assurance of reliability, safety, security, fairness, and interpretability of these systems is crucial to their successful deployment and, consequently, the future of our society. This is especially so in view of the impact of AI techniques on the design of CPS systems.
Our meticulously designed program prioritizes verification and validation as a major cross-cutting theme. We recognize the inherent challenges these twin pillars present at every phase of the Software Development Life Cycle (SDLC). From data collection and labelling to AI-centric system design, development, testing, and deployment – every stage demands rigorous validation and verification. Especially with data and learning emerging as critical components, the call for rigorous validation and verification is more pressing than ever.
Furthermore, our discussions will traverse the terrain of real-world fault tolerance, system optimization, and the often underplayed, yet vital aspect of human-machine interaction. We intend to highlight human involvement, not just as system overseers, but also in pivotal roles in system validation, interpretability, and in devising systems with nuanced, human-like reasoning capabilities.
This workshop is set to be a dynamic platform for knowledge exchange. It will draw together researchers from diverse disciplines, such as Software Engineering, Systems, Networking, Program Language, Machine Learning, Human Computer Interaction, Control Theory, Formal Verification, along with industry experts and AI enthusiasts worldwide. Attendees will have the opportunity to learn from global leaders in the field, engage in stimulating discussions, and collaborate on innovative solutions to enhance the trustworthiness of autonomous cyber-physical system.