Speaker Info
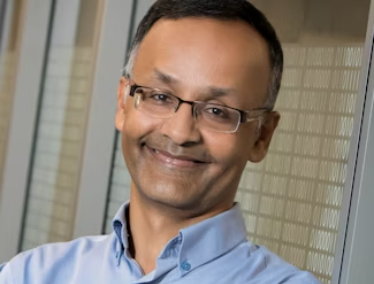
Professor Baruah joined Washington University in St. Louis in September 2017. He was previously at the University of North Carolina at Chapel Hill (1999-2017) and the University of Vermont (1993-1999). His research interests and activities are in real-time and safety-critical system design, scheduling theory, resource allocation and sharing in distributed computing environments, and algorithm design and analysis. He is a Fellow of the IEEE, and the recipient of the 2014 Outstanding Technical Contributions and Leadership Award of the IEEE Technical Committee on Real-Time Systems.
Abstract
Many modern safety-critical cyber-physical systems are characterized by highly dynamic workloads that repeatedly evolve as the system executes, thereby requiring that safety properties be repeatedly re-verified during run-time. Verification of many important safety properties is computationally highly intractable; it is therefore tempting to use Deep-Learning (DL) based techniques to classify system specifications according to whether they do or do not possess relevant safety properties. But how does one do so in a manner that does not compromise system safety despite the well-known fact that DL-based classification is prone to occasional errors? — this issue will be investigated in this presentation, with a particular focus on the verification of safety properties relating to timing correctness (i.e., schedulability).